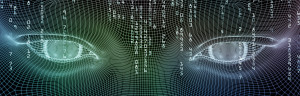
If there is one technology that promises to change the world more than any other over the next several decades, it is arguably machine learning. By enabling computers to learn certain things more efficiently than humans and discover certain things that humans cannot, machine learning promises to bring increasing intelligence to software everywhere and enable computers to develop ever new capabilities – from driving cars to diagnosing disease – that were previously thought impossible.
While most of the core algorithms that drive machine learning have been around for decades, what has magnified its promise so dramatically in recent years is the extraordinary growth of the two fuels that power these algorithms – data and computing power. Both continue to grow at exponential rates, suggesting that machine learning is at the beginning of a very long and productive run.
As revolutionary as machine learning will be, its impact will be highly asymmetric. While most machine learning algorithms, libraries and tools are in the public domain and computing power is a widely available commodity, data ownership is highly concentrated.
This means that machine learning will likely have a profound barbell effect on the technology landscape. On one hand, it will democratize basic intelligence through the commoditization and diffusion of services such as image recognition and translation into software broadly. On the other, it will concentrate higher-order intelligence in the hands of a relatively small number of incumbents that control the lion’s share of their industry’s data.
For startups seeking to take advantage of the machine learning revolution, this barbell effect is a helpful lens to look for the biggest business opportunities. While there will be many new kinds of startups that machine learning will enable, the most promising will likely cluster around the incumbent end of the barbell.
Democratization of Basic Intelligence
One of machine learning’s most lasting areas of impact will be to democratize basic intelligence through the commoditization of an increasingly sophisticated set of semantic and analytic services, most of which will be offered for free, enabling step-function changes in software capabilities. These services today include image recognition, translation and natural language processing and will ultimately include more advanced forms of interpretation and reasoning.
Software will become smarter, more anticipatory and more personalized, and we will increasingly be able to access it through whatever interface we prefer – chat, voice, mobile application, web, or others yet to be developed. Beneficiaries will include technology developers and users of all kinds.
This burst of new intelligent services will give rise to a boom in new startups that use them to create new products and services that weren’t previously cost effective or possible. Image recognition, for example, will enable new kinds of visual shopping applications. Facial recognition will enable new kinds of authentication and security applications. Analytic applications will grow ever more sophisticated in their ability to identify meaningful patterns and predict outcomes.
Startups that end up competing directly with this new set of intelligent services will be in a difficult spot. Competition in machine learning can be close to perfect, wiping out any potential margin, and it is unlikely many startups will be able to acquire data sets to match Google or other consumer platforms for the services they offer. Some of these startups may be bought for the asset values of their teams and technologies (which at the moment are quite high), but most will have to change tack in order to survive.
This end of the barbell effect is being accelerated by open source efforts such as OpenAI as well as by the decision of large consumer platforms, led by Google with TensorFlow, to open source their artificial intelligence software and offer machine learning-driven services for free, as a means of both selling additional products and acquiring additional data.
Concentration of Higher-Order Intelligence
At the other end of the barbell, machine learning will have a deeply monopoly-inducing or monopoly-enhancing effect, enabling companies that have or have access to highly differentiated data sets to develop capabilities that are difficult or impossible for others to develop.
The primary beneficiaries at this end of the spectrum will be the same large consumer platforms offering free services such as Google, as well as other enterprises in concentrated industries that have highly differentiated data sets.
Large consumer platforms already use machine learning to take advantage of their immense proprietary data to power core competencies in ways that others cannot replicate – Google with search, Facebook with its newsfeed, Netflix with recommendations and Amazon with pricing.
Incumbents with large proprietary data sets in more traditional industries are beginning to follow suit. Financial services firms, for example, are beginning to use machine learning to take advantage of their data to deepen core competencies in areas such as fraud detection, and ultimately they will seek to do so in underwriting as well. Retail companies will seek to use machine learning in areas such as segmentation, pricing and recommendations and healthcare providers in diagnosis.
Most large enterprises, however, will not be able to develop these machine learning-driven competencies on their own. This opens an interesting third set of beneficiaries at the incumbent end of the barbell: startups that develop machine learning-driven services in partnership with large incumbents based on these incumbents’ data.
Where the Biggest Startup Opportunities Are
The most successful machine learning startups will likely result from creative partnerships and customer relationships at this end of the barbell. The magic ingredient for creating revolutionary new machine learning services is extraordinarily large and rich data sets. Proprietary algorithms can help, but they are secondary in importance to the data sets themselves. The magic ingredient for making these services highly defensible is privileged access to these data sets. If possession is nine tenths of the law, privileged access to dominant industry data sets is at least half the ballgame in developing the most valuable machine learning services.
The dramatic rise of Google provides a glimpse into what this kind of privileged access can enable. What allowed Google to rapidly take over the search market was not primarily its PageRank algorithm or clean interface, but these factors in combination with its early access to the data sets of AOL and Yahoo, which enabled it to train its algorithms on the best available data on the planet and become substantially better at determining search relevance than any other product. Google ultimately chose to use this capability to compete directly with its partners, a playbook that is unlikely to be possible today since most consumer platforms have learned from this example and put legal barriers in place to prevent it from happening to them.
There are, however, a number of successful playbooks to create more durable data partnerships with incumbents. In consumer industries dominated by large platform players, the winning playbook in recent years has been to partner with one or ideally multiple platforms to provide solutions for enterprise customers that the platforms were not planning (or, due to the cross-platform nature of the solutions, were not able) to provide on their own, as companies such as Sprinklr, Hootsuite and Dataminr have done. The benefits to platforms in these partnerships include new revenue streams, new learning about their data capabilities and broader enterprise dependency on their data sets.
In concentrated industries dominated not by platforms but by a cluster of more traditional enterprises, the most successful playbook has been to offer data-intensive software or advertising solutions that provide access to incumbents’ customer data, as Palantir, IBM Watson, Fair Isaac, AppNexus and Intent Media have done. If a company gets access to the data of a significant share of incumbents, it will be able to create products and services that will be difficult for others to replicate.
New playbooks are continuing to emerge, including creating strategic products for incumbents or using exclusive data leases in exchange for the right to use incumbents’ data to develop non-competitive offerings.
Of course the best playbook of all where possible is for startups to grow fast enough and generate sufficiently large data sets in new markets to become incumbents themselves and forego dependencies on others, as for example Tesla has done for the emerging field of autonomous driving. This tends to be the exception rather than the rule, however, which means most machine learning startups need to look to partnerships or large customers to achieve defensibility and scale.
Machine learning startups should be particularly creative when it comes to exploring partnership structures as well as financial arrangements to govern them – including discounts, revenue shares, performance-based warrants and strategic investments. In a world where large data sets are becoming increasingly valuable to outside parties, it is likely that such structures and arrangements will continue to evolve rapidly.
Perhaps most importantly, startups seeking to take advantage of the machine learning revolution should move quickly, because many top technology entrepreneurs have woken up to the scale of the business opportunities this revolution creates, and there is a significant first-mover advantage to get access to the most attractive data sets.
This post was originally published on TechCrunch